Digital images can be obtained through a variety of sources including digital cameras. With rapidly increasing functionality and ease of use of image editing software, determining authenticity and identifying forged regions, if any, is becoming crucial for many applications. This paper presents methods for authenticating and identifying forged regions in digital photo images that have been acquired. Our reexamination of some of these recently successful experiments shows that variations in image clarity in the experimental datasets were correlated with authenticity, and may have acted as a confounding factor, artificially improving the results. To determine the extent of this factor’s influence on previous results. We demonstrate that a feature derived from Hidden-Markov-Tree-modeling of the digital photo image forgery using wavelet coefficients has the potential to distinguish copies from originals in the new dataset.
INTRODUCTION
Digital image forgery is the process of manipulating photographic images using image-processing tools like digital photo editing software to produce a digital image as evidence to the court; there is a need to identify the authenticity of the image. Digital Image forgery can be classified as the forgery with copy move and without copy move. In case of copy move type, some part of the image is cut and pasted somewhere in the image so that there are no manipulations like rotation, scaling etc. In the other case, due to the above-mentioned types, the data becomes highly correlated. The advent of the modern digital technology has not only brought about the prominent use of digital images in our daily activities but also the ease of creating image forgery using public accessible and user friendly image processing tools such as Photoshop. Hence the need for image authenticity assurance and detection of image forgery such as photomontage becomes increasingly acute as digital images takes role as news photographs, legal evidence and digital financial document.
A comparative study of the existing algorithms helps to investigate new methods. It opens up new avenues of research. It also helps the real world to overcome the problems being faced due to photomontage and forgery.
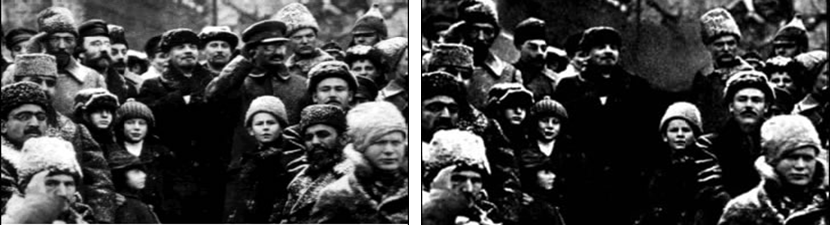
The literature survey has revealed that a substantial amount of work has been done in the field of digital image forgery and forensic science. Various algorithms and mathematical models were developed for detecting digital image forgery and various digital image forgery prevention methodologies, tools and techniques.
Manipulation of early photographic images was not an easy task, requiring a high level of technical expertise and specialized equipment. Alterations had to be made to the negatives, thus, if access could be obtained to the negatives, the authenticity or otherwise of the image could be determined by visual examination.
Tampering with photographic images dates back almost to the time when permanent photographic images were first created. One of the earliest instigators of photographic image tampering was Vladimir Ilyich Lenin, who, for political reasons, instructed that certain individuals be removed from photographs (Figure 1)